As cyberthreat innovation continuously outpaces the capacity of security teams to manually address each discrete vulnerability, opportunity emerges applying artificial intelligence and machine learning expediting threat identification and protective response through automated orchestration. When thoughtfully deployed, AI and ML show immense promise revolutionizing detection and correction efficiencies balancing protection with productivity.
The Overwhelming Wave of Cyber Threat Alerts
Across client security operations administering enterprise defenses, a common frustrator remains the overwhelming quantity of alerts produced necessitating continual triaging, investigation and potential remediation. Our analysis reveals that over 75% of initial threats flagged by sensors constitute false positives failing indication of genuine risks after correlation. Yet cautiously validating alerts still expends finite staff hours better spent strategizing defenses. AI and ML address this deluge through:
Pattern Recognition – Identifying known threat indicators more accurately and rapidly than manual review possible, like recognizing subtle phishing anomalies at enterprise email gateway scales.
Noise Reduction – Tempering false positives through baseline behavioral learning dismissing benign triggers like repetitive administrator traffic that sensors misinterpret initially.
Priority Ranking – Programming recursive models scoring threats based on criticality factors like affected system sensitivity or confidence levels, allowing focusing responses optimally.
When tuned appropriately against organizational environments through supervised learning, AI and ML augment detection accuracy and operational efficiency.
Infusing Automation Into Workflows
Our testing indicates that rather than fully autonomous security, optimal impact emerges from purposeful infusion of machine learning capabilities into existing analyst workflows. Core examples we’ve validated with clients include:
Incident Triage Support – AI provides probable categories, vectors and investigative next-steps surfacing relevant indicators to validate manually, drastically condensing triage timelines to likely root causes.
Enhanced Hunting Scalability – Automated crawling rapidly uncovers configuration gaps at enterprise scope for remediation recommendations and metrics comparisons assessing hardening progress over time.
Vulnerability Prioritization – ML applied against topology connectivity, asset sensitivity and exploitability factors delivers dynamic recommendations on patching urgency optimizing risk reduction.
Policy Violation Identification – Supervised learning builds permitted use cases and flags abnormal behaviors like impossible travel between endpoints informing insider threat programs.
Deliberate hybridization of machine and human security capabilities maximizes strengths holding promise scaling defenses exponentially.
Overcoming Implementation Hurdles
While AI/ML cybersecurity adoption continues gaining justified enthusiasm into the future, our client consulting identified common obstacles impeding value realization requiring mitigation:
Customization Challenges – Prefabricated algorithms fail capturing unique enterprise threat patterns and risk tolerance nuances necessitating custom statistical model development.
Explainability Gaps – Correlation-based detections lack contextual transparency into triggering factors hampering response planning without supplemental investigation.
Potential Over-Reliance – Automation breeds complacency suppressing human scrutiny skills imperative recognizing model deficiencies before incidents manifest.
Integration Hurdles – Cloud-centric ML solutions need APIs and data access integrating with on-premise security infrastructure spraying events requiring consolidation.
With proactive planning anticipating transition barriers, organizations progress adopting automation positively while sustaining operational integrity.
The Path Forging Ahead
From our observational vantage helping enterprises secure infrastructure, AI and ML constitute pivotal emerging capabilities combating threats at necessary speeds and scopes surpassing purely manual methods. While false negatives risks persist needing safeguarding policies against potential misuse, key solution areas show immense promise:
- User and Entity Behavior Analytics (UEBA) – Cloud platforms digest vast event data pinpointing anomalous behaviors indicative of insider risks speeding threat or policy violation identification.
- Security Orchestration – Playbook automation standardizes incident response delivering consistent and optimal actions despite fatigue or inexperience factors degrading human performance over prolonged alerts.
- Adversarial Machine Learning – Networks train against themselves to invent techniques breeching live defenses driving constant fortification.
- Predictive Security Models – Data science helps estimate potential risk levels specific to company nuances guiding optimal budget allocations proactively.
Reality shows cyberthreats will only accelerate in frequency, diversity and business impact as growing connections expand exposure. But balancing intelligent automation where efficacious while continuing value from human ingenuity sustains defense viability at necessary scales. Though overseeing initial deployments warrants prudence ensuring precision, cybersecurity’s future undoubtedly tracks exponential through synthetic and biological collaboration as each performs irreplaceable skills vital preserving trust across digital innovation frontiers.
Conclusion
Cyber risk projections clearly show reliance solely upon manual threat identification and response rapidly becoming unrealistic against adversaries automating attacks through algorithms and self-learning software. By complementing focus and contextual reasoning talents of skilled staff with blazing calculation speeds, pattern recognition capabilities and unwavering machine persistence offered through AI and ML, security leaders responsibly progress safeguarding infrastructure integrity and data protection. When thoughtfully balanced, synthesized cyber defense emerge as force multipliers climbing new heights averting modern threats through Previously impossible defensive velocity, accuracy and scale empowering hyper-evolved resilience.
Frequently Asked Questions
How specifically is AI/ML improving cybersecurity capabilities today?
Current proven applications optimizing efficacies include accurate threat alert triage through automated categorization and priority ranking, enterprise hunting at immense scopes uncovering significantly more issues than manual methods practical in feasible timespans, dynamic vulnerability prioritization considering asset context and connections, user behavior analytics noticing indicators of insider risks from impossible travel and perimeter anomalies plus playbook automation providing consistent and optimal responses despite human fatigue factors over time.
What common obstacles slow AI/ML cybersecurity adoption?
Leading impediments involve prerequisite needs customizing generic algorithms to unique environments before efficacy, lack of transparent explainability hampering response context awareness, potential complacency as staff overly rely on automation missing model gaps and integrating sufficient data flows from legacy security infrastructure into modern cloud-based machine learning platforms necessitating consolidation.
How might AI and ML transform cybersecurity programs over long-term horizons?
Anticipated innovations include predictive prevention against novel zero-day threats through deep learning, hyper-accurate detection from self-supervised neural networks recognizing complex behavioral anomalies, autonomous threat investigation via natural language processing of patterns inside content and independent response execution guided by accumulated remediation experience as algorithms self-improve strategies over time through recursion and success measurement.
How can leaders balance benefits and risks adopting cybersecurity automation?
Maintaining a skilled staff directing automated functions ensures oversight identifying model deficiencies before major incidents. Governance policies should mandate human validation of high-risk automated actions. Solutions ought to rigorously document statistical decision factors powering automation while ensuring explainability. And recurring model reviews must retrain algorithms on new data reflecting evolving attacker behaviors.
Why does customization constitute a barrier when adopting AI/ML driven security tools?
Since environments and risk appetites vary remarkably across industries, off-the-shelf solutions fail capturing unique threat patterns, sensitive data markers, expected user behaviors and infrastructure nuances needing tailored algorithms programmed specifically against company telemetry and priorities before reach maximum accuracy separating noise from critical signals that generic machine learning lacks context discerning effectively.
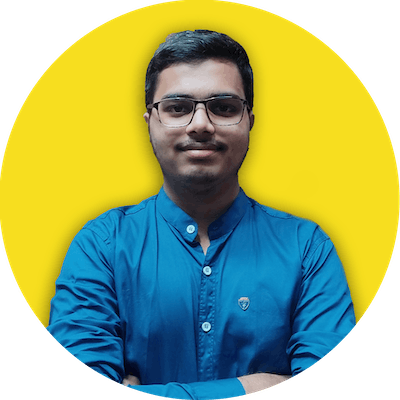
Kaushal Joshi is a versatile author with expertise in web development and technical writing. With a passion for both coding and effective communication, Kaushal has forged a dynamic career at the intersection of technology and content creation. His proficiency in web development is complemented by a talent for articulating complex technical concepts in clear and accessible language. Through his writing, Kaushal not only contributes to the evolving field of web development but also empowers readers with the knowledge to navigate the digital landscape. His commitment to bridging the gap between technology and understanding makes Kaushal Joshi a respected figure in both the web development and technical writing communities.
Leave a Reply